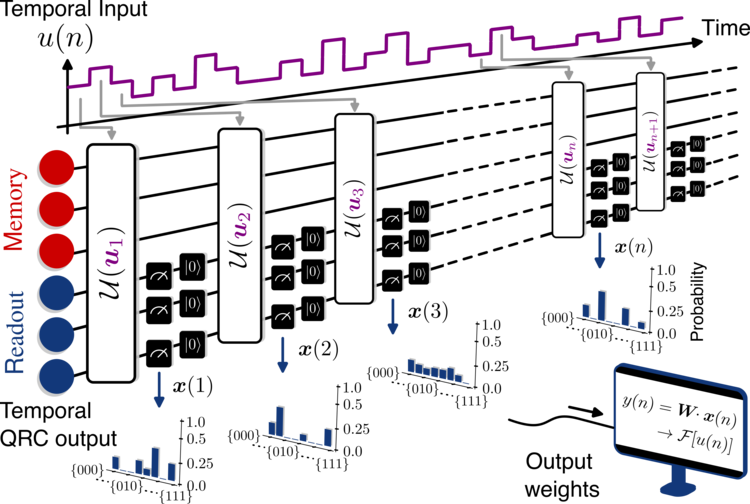
Overcoming the Coherence Time Barrier in Quantum Machine Learning on Temporal Data
Type
The practical implementation of many quantum algorithms known today is limited by the coherence time of the executing quantum hardware and quantum sampling noise. Here we present a machine learning algorithm, NISQRC, for qubit-based quantum systems that enables inference on temporal data over durations unconstrained by decoherence. NISQRC leverages mid-circuit measurements and deterministic reset operations to reduce circuit executions, while still maintaining an appropriate length persistent temporal memory in the quantum system, confirmed through the proposed Volterra Series analysis. This enables NISQRC to overcome not only limitations imposed by finite coherence, but also information scrambling in monitored circuits and sampling noise, problems that persist even in hypothetical fault-tolerant quantum computers that have yet to be realized. To validate our approach, we consider the channel equalization task to recover test signal symbols that are subject to a distorting channel. Through simulations and experiments on a 7-qubit quantum processor we demonstrate that NISQRC can recover arbitrarily long test signals, not limited by coherence time.