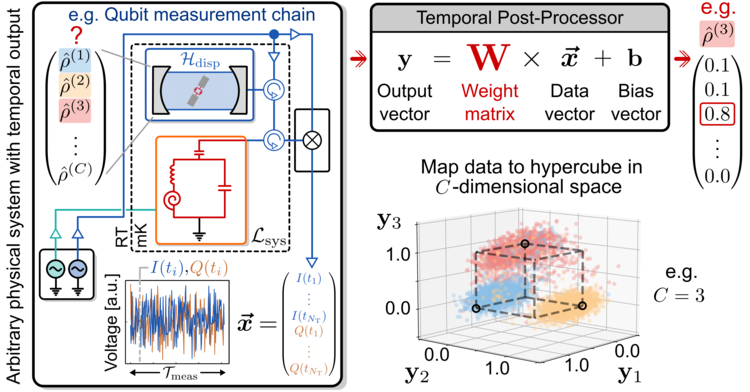
Practical trainable temporal post-processor for multi-state quantum measurement
Type
We develop and demonstrate a trainable temporal postprocessor (TPP) harnessing a simple but versatile machine learning algorithm to provide optimal processing of quantum measurement data subject to arbitrary noise processes for the readout of an arbitrary number of quantum states. We demonstrate the TPP on the essential task of qubit state readout, which has historically relied on temporal processing via matched filters in spite of their applicability for only specific noise conditions. Our results show that the TPP can reliably outperform standard filtering approaches under complex readout conditions, such as high-power readout. Using simulations of quantum measurement noise sources, we show that this advantage relies on the TPP’s ability to learn optimal linear filters that account for general quantum noise correlations in data, such as those due to quantum jumps, or correlated noise added by a phase-preserving quantum amplifier. Furthermore, we derive an exact analytic form for the optimal TPP weights: this positions the TPP as a linearly scaling generalization of matched filtering, valid for an arbitrary number of states under the most general readout noise conditions, all while preserving a training complexity that is essentially negligible in comparison with that of training neural networks for processing temporal quantum measurement data. The TPP can be autonomously and reliably trained on measurement data and requires only linear operations, making it ideal for field-programmable gate array implementations in circuit QED for real-time processing of measurement data from general quantum systems.